Cutting through the AI, ML hype Two experts share exactly where the security industry is with this technology
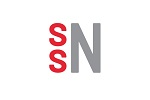
By Ginger Hill
Updated Wed February 26, 2020
YARMOUTH, Maine—Future possibilities for Artificial Intelligence (AI) and Machine Learning (ML) are endless and stretch as far as the imagination can go, and continue to be hot topics of discussion among security professionals. While it's smart to glimpse into the future, the security industry has to make “living in reality” a top priority, especially when meaning and current use cases for AI and ML tend to come with an air of confusion.
Defining exactly and precisely what each is, and what both can currently be used for right now, is extremely valuable for the security industry to help mitigate confusion, for security professionals for business continuity and for end users to ensure safety and security for all.
Defining AI and ML
According to Stuart Tucker, vice president, Enterprise Solutions, AMAG Technology, AI is “any process where a machine or computer is programmed to think and/or act like a person whereas machine learning is where a system is capable of looking at past events and changes its behavior based on what it learned from the results.”
Taking a historical view point, Jim Shaw, Crystal Group's executive vice president of engineering, defined AI as “using a machine/computer to simulate a capability or emulate a biological process associated with human thought.” Shaw believes that is what John McCarthy, a mathematics professor, was contemplating in 1956 at Dartmouth College when he coined the term. However, Shaw admitted that his definition of AI is less ambitious and more simplified. “I think AI, as it is more commonly used today, is the compute capability to quickly sort through large amounts of data and to look for specific patterns, using algorithms designed to compare and contrast differences.”
As for ML, Shaw thinks it is a subset of AI in which an algorithm is provided feedback, assessing how well the algorithm worked on a data set by determining if the right answer was achieved.
“This feedback loop modifies the parameters of the algorithm so the system changes how it identifies the next pattern,” explained Shaw. “Technically, it changes the weighting factors of the neural network layers to improve the accuracy of the last answer. Bottom line, the feedback/correction loop is the 'learning' part of ML.”
Adopting a standard industry definition for AL and ML would be a huge step in clarifying and teaching correct meanings across the industry so that all security professionals are on the same page, so to speak. “There certainly needs to be a common lexicon, which implies common understanding of these terms,” said Shaw.
Tucker looks at a standard definition in terms of clarifying what a system does to end users. “If you really dig down deeply, even self-learning systems are based on algorithms and programming at some level which they use to 'train' behavior,” he explained. “When you look at it from a very granular level, it can get confusing as to what is really deserving of being called AI/ML versus programmed behavior in some cases.”
There's no doubt that these two concepts are difficult and adding to the confusion is other “vocabulary” words—neural networks, quantum computing, cryptology, etc.—that seem to play into AI and ML, in addition to misinformation, further convoluting meanings and conceptions. Perhaps for the sake of argument and for this article, we can at least all agree that AI is the process and ML is the capability of the process.
Actual trends and real use cases
The security industry is currently witnessing the use of AI and ML to quickly consume, process and analyze data to predict possible risks. AI and ML is used to look for anomalies and elevated risk.
“People tend to be creatures of habit,” Tucker said. “When a person starts doing things that are out of the ordinary for them, it could point to an elevated risk and is at least worth noticing.”
Focusing specifically on human behavior through the lens of AI and paying attention to physical human behavior can provide the needed information to make relevant security decisions, such as “helping your staff make decisions about an identity's access to physical and logical assets,” said Tucker. “Should they be granted access to a data center or the C-Suite? Do I allow them to log in at a location when they have not badged into the building?”
Also trending with AI and ML is more compute being required farther away from the data center core, while at the same time, network speeds are increasing.
“Network interface speeds are increasing from 10GbE to 200GbE, indicating the amount of data is growing significantly,” Shaw said. “As IoT and 5G continue to come into play, we can expect the number of devices used for security to exponentially increase, making it more critical to push the right data — and a lot of it.”
Shaw also said that we are currently seeing AI used for retinal scans, facial recognition and finger printing in high-risk places such as court rooms, airports, train stations and large sporting arenas.
“I think AI is a great way for us to sort and sift through large amounts of data to look for key pieces of information that are, frankly, boring and mundane tasks for the average person—and in many cases, beyond what humans are even capable of,” Shaw said.
The security industry is currently seeing and participating in the infancy of AI and ML or as Tucker puts it, “just starting to scratch the surface,” however, as this technology matures, “we will be able to actually pre-detect and prevent issues based on recognized patterns of bad behaviors and the learned outcomes that have occurred in the past,” he concluded.
Comments